Synthetic data and simulation: The Key duo in the future of the AI era
- AI
- 8 mins
The development of Artificial Intelligence (AI) has ushered in a new era of data-driven decision-making. As AI continues to progress, there is an increasing demand for high-quality data to fuel the development of intelligent algorithms. However, real-world data often come with limitations, such as the potential for bias, incomplete data, or privacy concerns.
Synthetic data and simulation have emerged as two powerful tools to address these limitations and unlock the full potential of AI. In this blog post, we will explore the definitions, benefits, and use cases of synthetic data and simulation, and examine predictions for the future of these technologies from Gartner.
Synthetic Data: Definition and Benefits
What is Synthetic Data?
In simple terms, it is artificially generated data that is designed to mimic the statistical properties of real-world data. It is often used in machine learning applications to train models without exposing sensitive or confidential information.
Think of synthetic data as a lab-created protein with specific functions and properties. Just as synthetic protein is manufactured artificially in industries to imitate the structure and role of natural proteins, synthetic data is generated artificially to mimic the statistical properties and relationships of real-world data.
What are the benefits of synthetic data?
- Provides a solution for small or incomplete real-world datasets.
- Enables quick and easy data generation without time-consuming data collection and labeling.
- Allows simulation of rare or extreme scenarios difficult to capture in real-world data.
- Offers customization and manipulation for testing and evaluating machine learning models.
- Safeguards the privacy and security of sensitive information.
- Reduces bias in machine learning models by creating balanced datasets.
- Augments real-world data by adding samples or variations.
- Lowers costs associated with collection and labeling, and mitigates risks and ethical concerns of using real-world data.
Use cases of synthetic data
From retail to healthcare, synthetic data is one of the most promising developments in the field of AI, with numerous applications in areas such as customer research, healthcare, and autonomous driving.
Synthetic Data in Customer Research
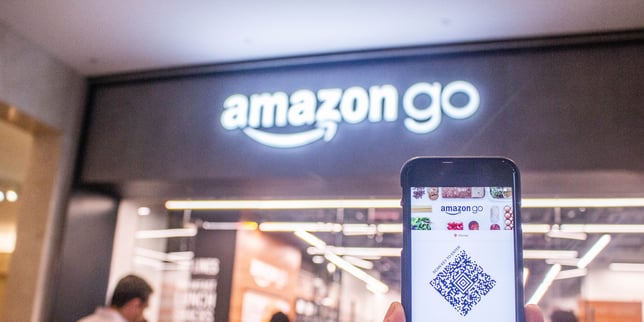
Amazon Go, the cashierless store operated by Amazon, uses synthetic data to train its machine learning algorithms without compromising the privacy of its customers. The algorithms are trained to recognize and track customer movements and actions, as well as to detect and track products as they are taken from shelves and placed in shopping carts.
Synthetic Data in Healthcare
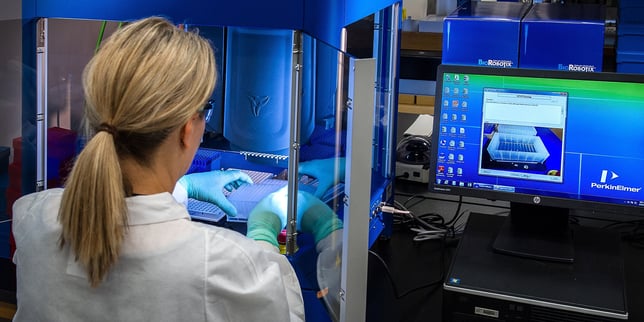
According to the article "The promises and challenges of using synthetic data for medical imaging" published in the journal Nature Biomedical Engineering, a potential use of synthetic data in healthcare would allow training and validating machine learning models for medical imaging tasks, such as identifying tumors or classifying diseases.
Synthetic Data in Autonomous Driving:
Another example of the use of synthetic data is in the field of autonomous driving, where synthetic data is used to train machine learning models to recognize and respond to various driving scenarios and conditions, such as weather, lighting, and traffic patterns that would be difficult to predict.
Simulation: Definition and Benefits
What is simulation?
Simulation is a process of creating a model of a real-world system or phenomenon using a computer program or other mathematical techniques. The model is designed to imitate or replicate the behavior of the real-world system, allowing researchers or engineers to study the system under different conditions or scenarios.
Its goal is to create a virtual environment that behaves like the real system as closely as possible, allowing researchers to study and test the system without the risks or costs associated with physical experimentation.
Benefits of simulation
- Allows researchers to study and test systems in a virtual environment, reducing the risk of accidents or harm that could occur during physical experimentation.
- Provides a cost-effective alternative to physical experimentation, reducing the need for expensive equipment, materials, and resources.
- Generates highly accurate results, allowing researchers to study and predict the behavior of systems under a wide range of conditions.
- Delivers greater flexibility in the types of experiments that can be conducted.
- Provides researchers with a deeper understanding of complex systems or phenomena, allowing for better decision-making and problem-solving.
- Can be used to study the impact of systems on the environment without actually affecting it, reducing the environmental cost of research and experimentation.
Use Cases of Simulation
Simulation is a powerful tool that can be applied to a variety of industries and scenarios, from optimizing processes in factories to improving traffic flow in entire cities.
Simulation in the Development of Smart Cities
.jpg?width=644&height=362&name=zhu-hongzhi-tzFVsg6nWtM-unsplash%20(2).jpg)
Digital twins, a form of simulation, can be combined with synthetic data to simulate the production process, allowing manufacturers to optimize efficiency and reduce waste. As digital twins have proved to be effective in optimizing processes and efficiencies in factories, hotels, and wind farms, it begs the question: can they be applied to entire cities?
Singapore and Shanghai have already implemented complete digital twins that focus on improving energy consumption, traffic flow, and urban development planning. This trend of creating smart cities is rapidly becoming a reality, offering a promising approach to reducing pollution and enhancing the quality of life for residents.
Simulation in Autonomous Driving
Simulation can be used to generate synthetic data that complements real-world datasets in training perception systems for autonomous cars. With advancements in computer graphics technology, self-driving car simulators like DeepGTA-V and CARLA can create highly realistic driving environments and generate large amounts of training data.
It can also generate dangerous scenarios that are difficult or impossible to recreate in the real world, such as severe weather or rare accident scenarios.
Simulation in Manufacturing
.jpg?width=644&height=430&name=carlos-aranda-QMjCzOGeglA-unsplash%20(2).jpg)
By using simulation in manufacturing, engineers can better understand real-world problems safely and efficiently by providing a strong dataset from which they can make informed decisions. This can diminish months of physical testing of components into a few seconds, and when combined with AI systems, it can save money on labor and cut time even further. This balance of AI and simulation technologies is vital to the future of engineering design and manufacturing.
The Future of Synthetic Data and Simulation: Predictions from Gartner
By 2024, the use of synthetic data created with generative AI will halve the volume of real data needed for machine learning.
By using synthetic data, which is generated by AI algorithms rather than collected from the real world, organizations can reduce their reliance on expensive and time-consuming data collection efforts.
By 2027, data science organizations will cut AI technical debt by 70% by using simulation platforms and technologies to manage the complexity of AI systems
By simulating different scenarios and testing AI models in a virtual environment, organizations can identify potential issues before deploying AI systems in the real world, reducing the risk of costly errors and improving the overall reliability of AI solutions.
By 2030, most AI models will be trained in simulated environments.
Training AI models in the real world can be expensive and time-consuming, and it can also be risky if the models are not sufficiently robust. Gartner predicts that by 2030, most AI models will be trained in simulated environments, where they can be tested and refined in a safe and controlled environment.
Challenges and Limitations of Synthetic Data and Simulation
While this approach has its advantages, it also has limitations and challenges that must be taken into account when using it in machine-learning applications. For example:
- Lack of real-world complexity: synthetic data generated using algorithms may not capture the complexity and nuance of real-world data, leading to oversimplified or unrealistic datasets.
- Overfitting: synthetic data can be prone to overfitting, resulting in biased models that do not perform well on new data.
- Limited scope: synthetic data is limited by the scope of the data that was used to generate it, which can result in a narrow range of data.
- Privacy concerns: synthetic data may still contain identifiable information, raising privacy concerns that may require additional measures to protect sensitive information.
- Cost and time: generating high-quality synthetic data can be a time-consuming and costly process, especially for large amounts of data.
- Evaluation: it can be challenging to evaluate the quality of synthetic data since there is no ground truth to compare it to, making it difficult to assess the performance of machine learning models trained on synthetic data.
Conclusion
In conclusion, synthetic data and simulation are two powerful tools that are transforming the way we develop and implement AI. Synthetic data is used to overcome the limitations of real-world data and generate large amounts of data that are customized and free from privacy concerns.
On the other hand, simulation allows researchers and engineers to study and test systems in a virtual environment, reducing the risks and costs associated with physical experimentation. The benefits of these technologies are many, including improved accuracy, reduced costs, and enhanced decision-making.
The use cases of synthetic data and simulation are also vast, ranging from customer research to autonomous driving to smart cities. As AI continues to advance, the use of synthetic data and simulation will likely become even more prevalent, allowing for even greater innovation and development in the field. However, it is important to keep in mind the potential ethical and social implications of these technologies and to use them responsibly.